April 28, 2020 / by Giorgia Cantisani / UGOSA
User-guided one-shot deep model adaptation for music source separation
Music source separation is the task of isolating individual instruments which are mixed in a musical piece. This task is particularly challenging, and even state-of-the-art models can hardly generalize to unseen test data. Nevertheless, prior knowledge about individual sources can be used to better adapt a generic source separation model to the observed signal.
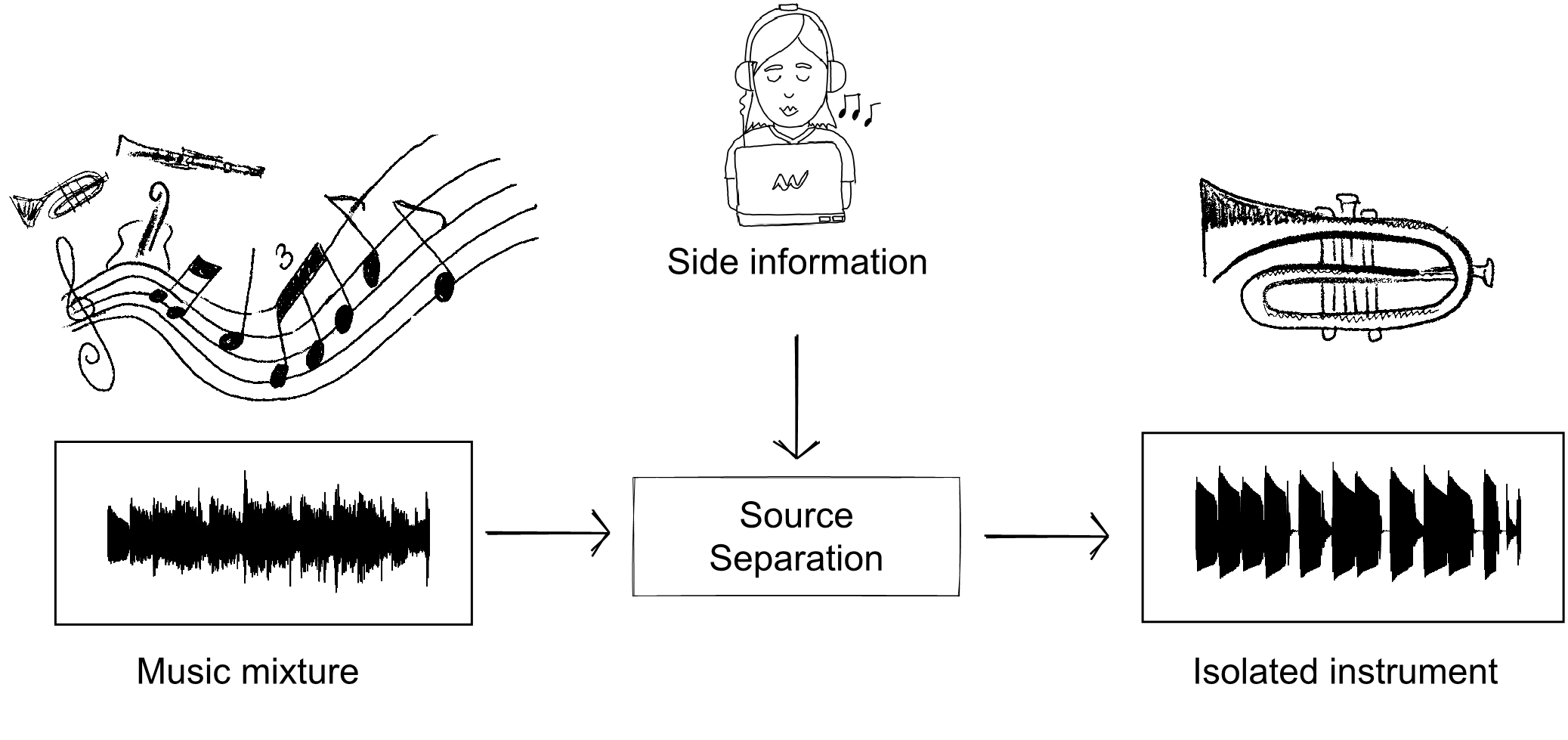
In this work, we propose to exploit a temporal segmentation provided by the user, that indicates when each instrument is active, in order to fine-tune a pre-trained deep model for source separation and adapt it to one specific mixture. This paradigm can be referred to as user-guided one-shot deep model adaptation for music source separation, as the adaptation acts on the target song instance only. The adaptation is made possible thanks to a proposed loss function which aims to minimize the energy of the silent sources while at the same time forcing the perfect reconstruction of the mixture.
Our results are promising and show that state-of-the-art source separation models have large margins of improvement especially for those instruments which are underrepresented in the training data. Below you can find some audio examples from the MUSDB18 test set.
OTHER
AM Contra - Heart Peripheral
Bobby Nobody - Stitch Up
BASS
Buitraker - Revo X
Cristina Vane - So Easy
DRUMS
Arise - Run Run Run
Angels In Amplifiers - I'm Alright
VOCALS
Ben Carrigan - We'll Talk About It All Tonight
Buitraker - Revo X
For more details, please refer to the paper User-guided one-shot deep model adaptation for music source separation by Cantisani G. et al..
This project was conducted during my internship in InterDigital while pursuing my PhD Thesis at Télécom Paris under the supervision of Alexey Ozerov, Slim Essid and Gaël Richard.
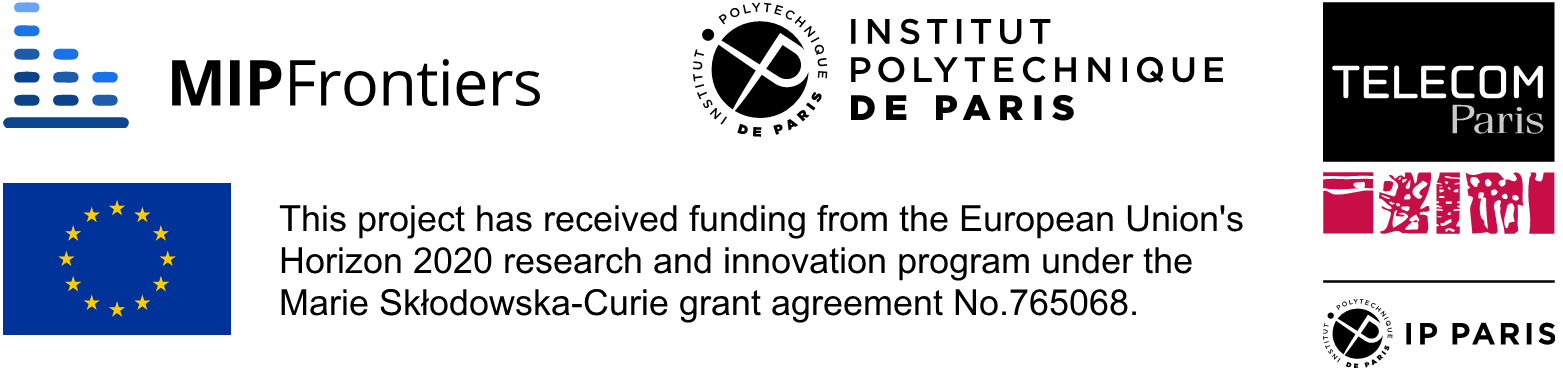
